主题笔记_AI绘画
1 工具使用
1.1 Midjourney vs Stable Diffusion
收费、审核、门槛;开源、部署、海量模型、可控、要求
生态:平台、插件、模型、文档;自已训练
1.2 环境
docker 镜像:siutin/stable-diffusion-webui-docker
1 | $ nvidia-docker run -it --name sdw --gpus all --network host \ |
1.3 模型
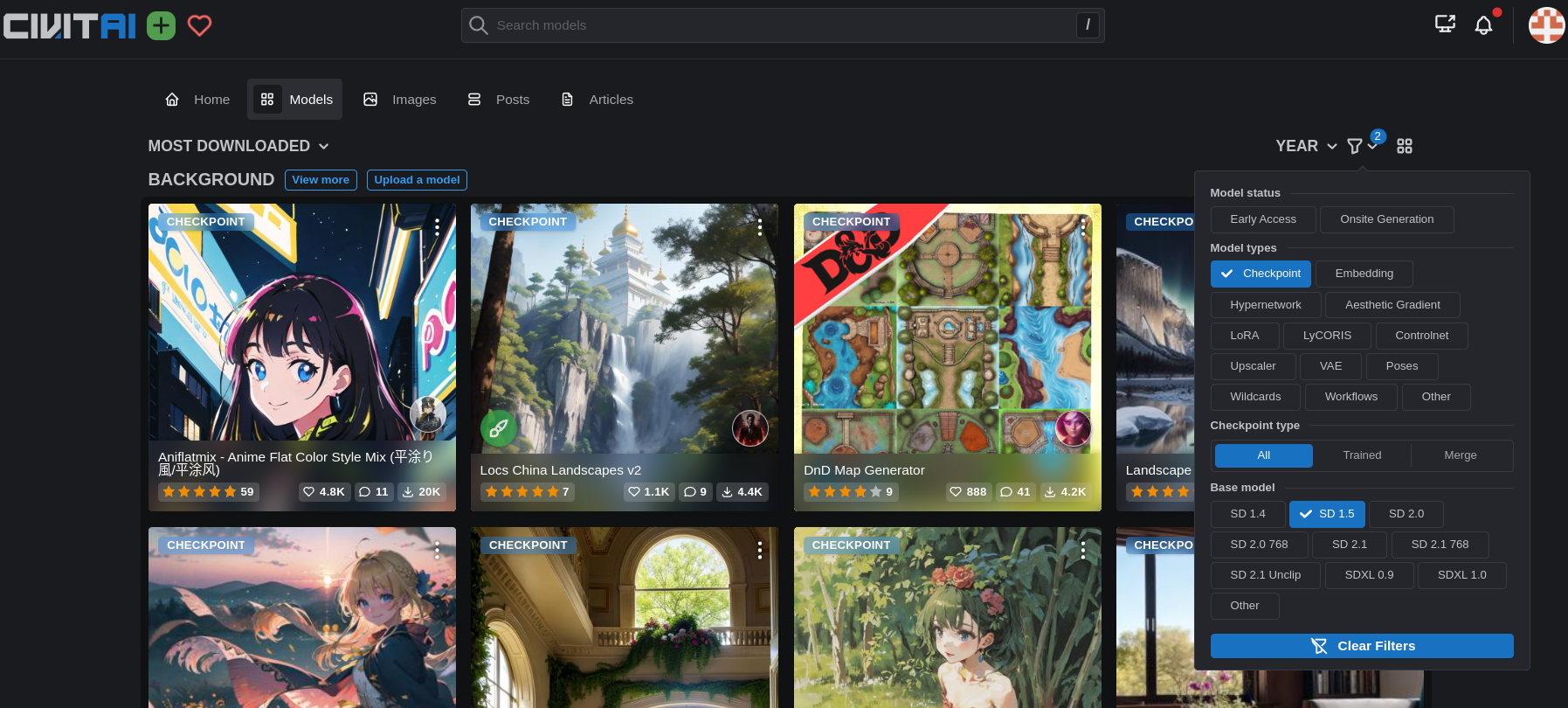
- 基本模型:SDXL 1.0,anything
- LoRA 模型:Detail Tweaker LoRA,GHIBLI_Background
- VAE 模型:2d/真实
- ControlNet 模型
- CLIP
1.4 界面操作
2 底层技术
2.1 U-Net
基础技术 U-Net: Convolutional Networks for Biomedical Image Segmentation
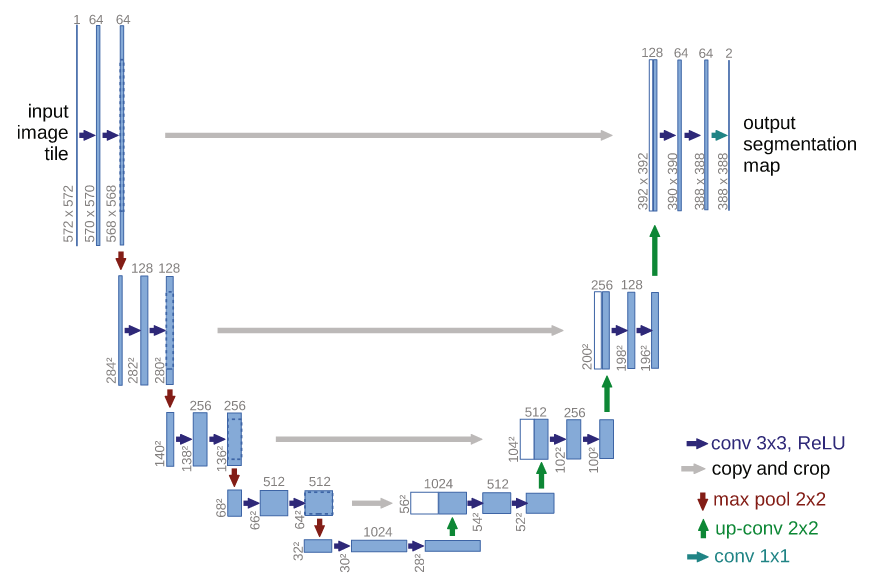
\[ w(\mathbf{x})=w_{c}(\mathbf{x})+w_{0} \cdot \exp \left(-\frac{\left(d_{1}(\mathbf{x})+d_{2}(\mathbf{x})\right)^{2}}{2 \sigma^{2}}\right) \]
2.2 VAE
基础技术 VAE:Auto-Encoding Variational Bayes
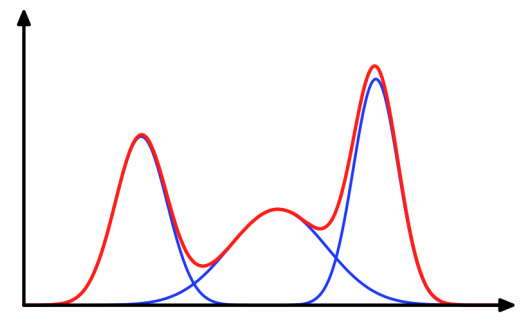
2.2.1 变分推断
变分 Variational 是通过引入一个简化的参数化分布来近似复杂的后验分布。这个参数化分布被称为变分分布,它属于一种可计算的分布族。通过调整变分分布的参数,使其尽可能接近真实的后验分布,从而实现近似推断。
2.2.2 变分下界
变分下界(variational lower bound)通常用于衡量变分分布与真实后验分布之间的差异。
\[ ELBO = E[log\ p(x, z) - log\ q(z)] \]
其中,ELBO 代表变分下界(Evidence Lower BOund),x 代表观测数据,z 代表未知变量,p(x, z) 表示真实的联合分布,q(z) 表示变分分布。
3 扩散模型进化
3.1 DM
扩散模型开山之作 DM Deep Unsupervised Learning using Nonequilibrium Thermodynamics
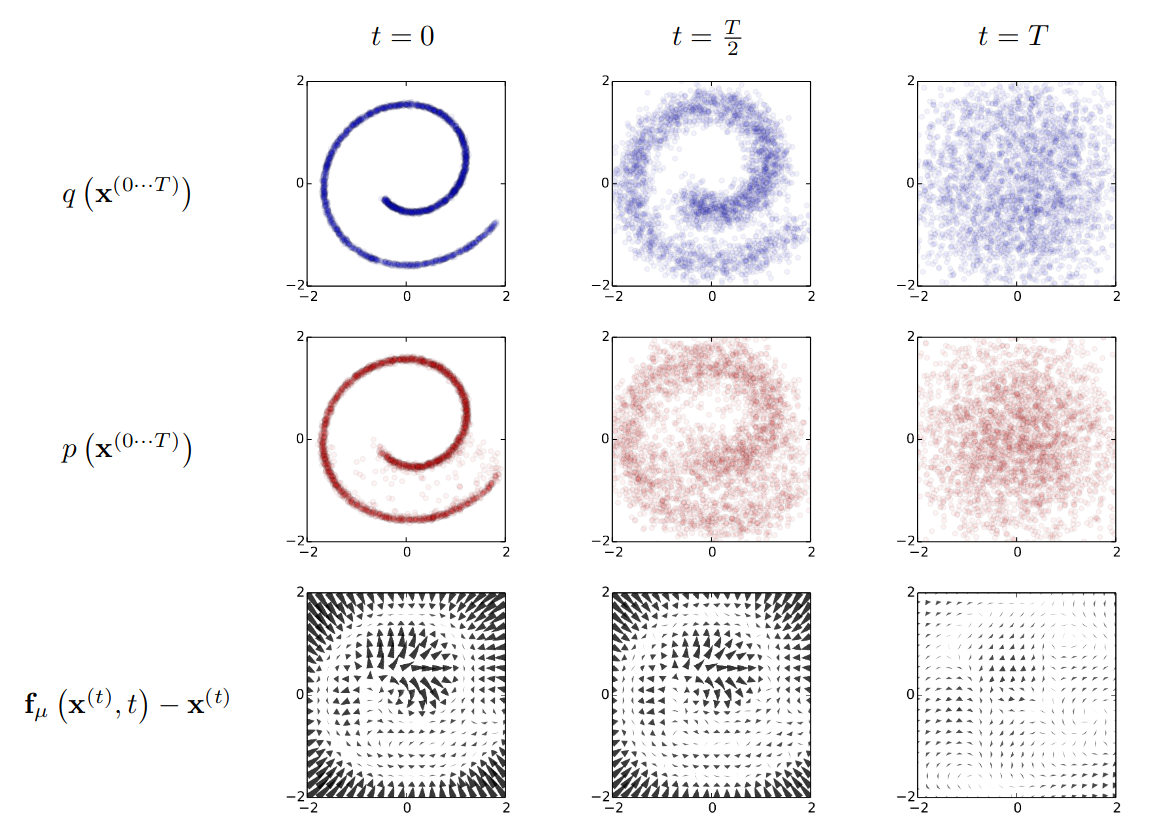
3.2 DDPM
扩散概率模型用于高质量的图像合成 DDPM:Denoising Diffusion Probabilistic Models
\[ 0<\beta_1<\beta_2<...<\beta_T<1 \] \[ p_\theta (\mathbf{x}_{t-1} | \mathbf{x}_t) = \mathcal{N}(\mathbf{x}_{t-1}; \mu_\theta(\mathbf{x}_{t},t), \Sigma_\theta (\mathbf{x}_{t},t)) \] \[ q(\mathbf{x}_t | \mathbf{x}_0) = \cal{N}(\mathbf{x}_t; \sqrt{\bar{\alpha}_t} \mathbf{x}_0, (1- \bar{\alpha}_t) \mathbf{I}) \] \[ \| \mathbf{\epsilon} - \mathbf{\epsilon}_\theta(\mathbf{x}_t, t) \|^2 = \| \mathbf{\epsilon} - \mathbf{\epsilon}_\theta( \sqrt{\bar{\alpha}_t} \mathbf{x}_0 + \sqrt{(1- \bar{\alpha}_t) } \mathbf{\epsilon}, t) \|^2. \]
3.3 LDM
模型提速 LDM:High-Resolution Image Synthesis with Latent Diffusion Models
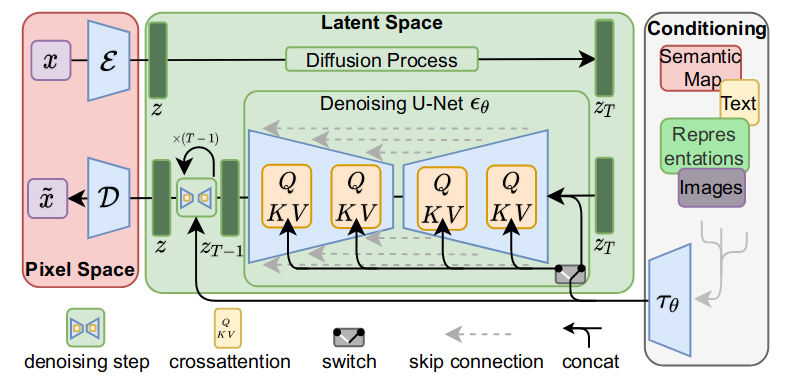
3.4 SDXL
最新 AI 绘画大模型 SDXL: Improving Latent Diffusion Models for High-Resolution Image Synthesis
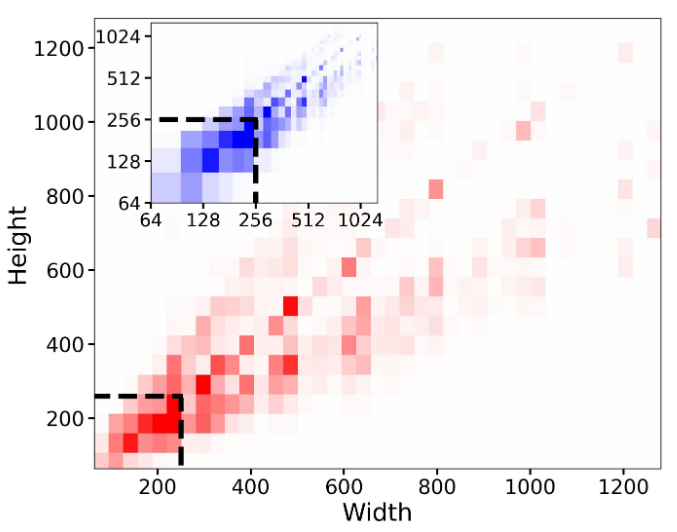
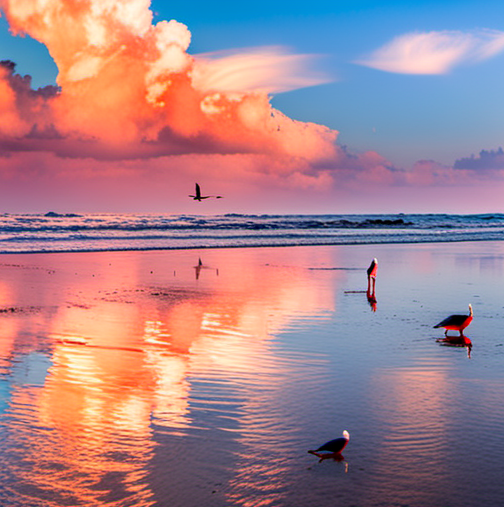
4 周边技术
4.1 LoRA
用小模型扩展大模型 LoRA: Low-Rank Adaptation of Large Language Models
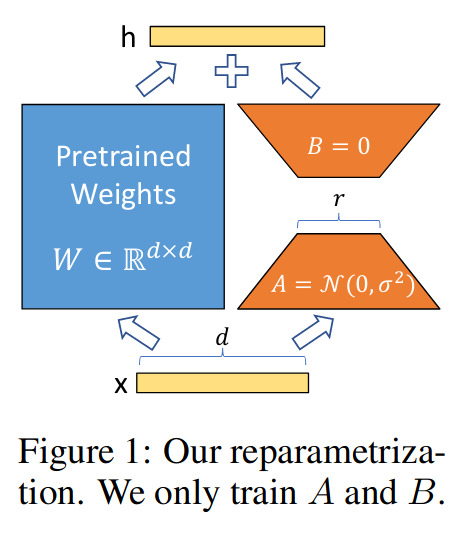
4.2 ControlNet
精准控制 ControlNet 绘图:Adding Conditional Control to Text-to-Image Diffusion Models
\[ y_c = F(x;\theta)+Z(F(x + Z(c;\theta_{z1});\theta_c);\theta_{z2}) \]